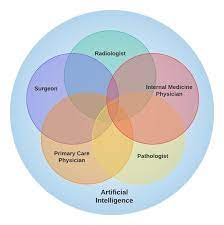
Multimodal AI
Multimodal AI
Important for Prelims Exams:
Open AI, Chat GPT-4, AI, Unimodal AI, Multimodal AI
Important for Mains Exams:
Multimodal Artificial Intelligence, Its Applications and Significance
October 12, 2023
In News:
- Open AI recently announced the launch of its most advanced AI, GPT-4, as a multimodal AI.
- It will be able to more easily process and understand pictures, sounds and other forms of data, meaning it is more capable than previous versions of GPT.
Multimodal Artificial Intelligence:
Introduction:
- Multimodal AI is an advanced form of artificial intelligence that can analyze and interpret data in multiple ways simultaneously.
- It is capable of more accurate and human-like reasoning and decision making.
Unimodal AI vs Multimodal AI:
- The fundamental difference between multimodal AI and unimodal AI is the use of data.
- Unimodal AI is designed to work with a single source or type of data. For example, a unimodal AI system like Chat GPT uses natural language processing (NLP) algorithms to understand text content and extract meaning from it, and the chat Bot can only generate text output. Unimodal AI is tailored to a specific task.
- Multimodal AI processes data from multiple sources, including video, images, speech, sound, and text, enabling more detailed and nuanced perceptions of a particular environment or situation.
- Multimodal AI more closely simulates human perception and increases the accuracy of AI systems.
- e.g., Seamless M4T, launched by Meta, is a multimodal AI translation and transcription model that is capable of performing various tasks including speech-to-text, speech-to-speech, text-to-speech, and text-to-text translations.
Applications of Multimodal AI:
- Virtual assistants: Multimodal AI can be used to create virtual assistants that can understand and respond to natural language commands.
- Audio Processing: Multimodal AI models can be used to understand the content of audio. It can be used for tasks such as speech recognition, speaker identification, and music classification.
- Healthcare: Multimodal AI can help improve medical imaging analysis, disease diagnosis, personalized treatment planning. For example, by combining medical images with patient records and genetic data, healthcare providers can gain a more accurate understanding of a patient's health.
- Retail: In retail, it can be used to enhance customer experience and increase sales. Using user behavior data, product images and customer reviews, retailers can provide personalized recommendations and customize product searches.
- Agriculture: Multimodal AI can help monitor crop health, predict yields, and optimize farming practices. By integrating satellite imagery, weather data and soil sensor data, farmers can gain deeper insight into crop health and optimize irrigation and fertilizer application.
- Manufacturing: Multimodal AI can be leveraged to improve quality control, predictive maintenance, and supply chain optimization.
- Robotics: Multimodal AI is central to robotics development using which robots can successfully interact with real-world environments.
- Entertainment: Multimodal AI algorithms can be used to extract features about emotions, speech patterns, facial expressions and actions that can create targeted content for specific demographics.
- Computer Vision: Multimodal AI models can be used to understand the content of pictures and videos. It can be used for tasks like object detection, scene understanding and facial recognition.
- Self-driving cars: Multimodal AI can be used to power self-driving cars that can see, hear, and understand the world around them.
Significance of Multimodal AI:
- Better accuracy: Multimodal AI models can often achieve higher accuracy than single-modality models. This is because they are able to use multiple data sources to inform their predictions.
- Enhanced robustness: Multimodal AI models are often more robust to noise and errors than single-modality models. This is because they are able to use multiple data sources to compensate for missing or corrupted data.
- Advanced understanding: Multimodal AI models can use multiple data modalities to gain a more comprehensive understanding of the world around them. It can be used for tasks such as understanding the context of a conversation or the meaning of a visual scene.
- Data Aspects of Multimodal AI: One of the most important aspects of Multimodal AI is data. Multimodal AI models need to be trained on large datasets of multimodal data to learn how to effectively process and understand information from different sources. This data can be collected from a variety of sources, such as social media, sensor data, and medical records.
- Natural Language Processing (NLP): Multimodal AI models can be used to understand the meaning of text as well as the context in which it is used. It can be used for tasks such as machine translation, text summarization, and question answering.
Challenges related to multimodal AI:
- Data collection: The data sets required to conduct multimodal AI include a large variety of data (text, images, audio, video). Storing and processing such data volumes can be quite expensive.
- Data integration: Combining and synchronizing different types of data can be challenging because data from multiple sources will not have the same format. Ensuring seamless integration of multiple modalities and maintaining consistent data quality can be difficult and time-consuming.
- Data Bias: Data bias and maintaining data integrity can be a problem in training AI models.
- The data used to train multimodal AI models needs to be carefully curated to ensure that it is accurate and representative of the real world. The data also needs to be labeled, which means it must be tagged with the correct information about the contents of the data. This labeling process can be time-consuming and expensive, but it is essential for the accuracy of multimodal AI models.
- Despite these challenges, multimodal AI is a promising area of research that has the potential to revolutionize the way AI is used. As the amount of multimodal data continues to grow, multimodal AI models will become increasingly powerful and versatile.
Conclusion:
- Multimodal AI is still a relatively new area of research, but it has the potential to revolutionize the way AI is used. By combining the strengths of different data modalities, multimodal AI models can be created to create more powerful and versatile AI systems.
--------------------------------
What is Multimodal Artificial Intelligence? Write about its applications and significance.